What are the environmental impacts of AI?
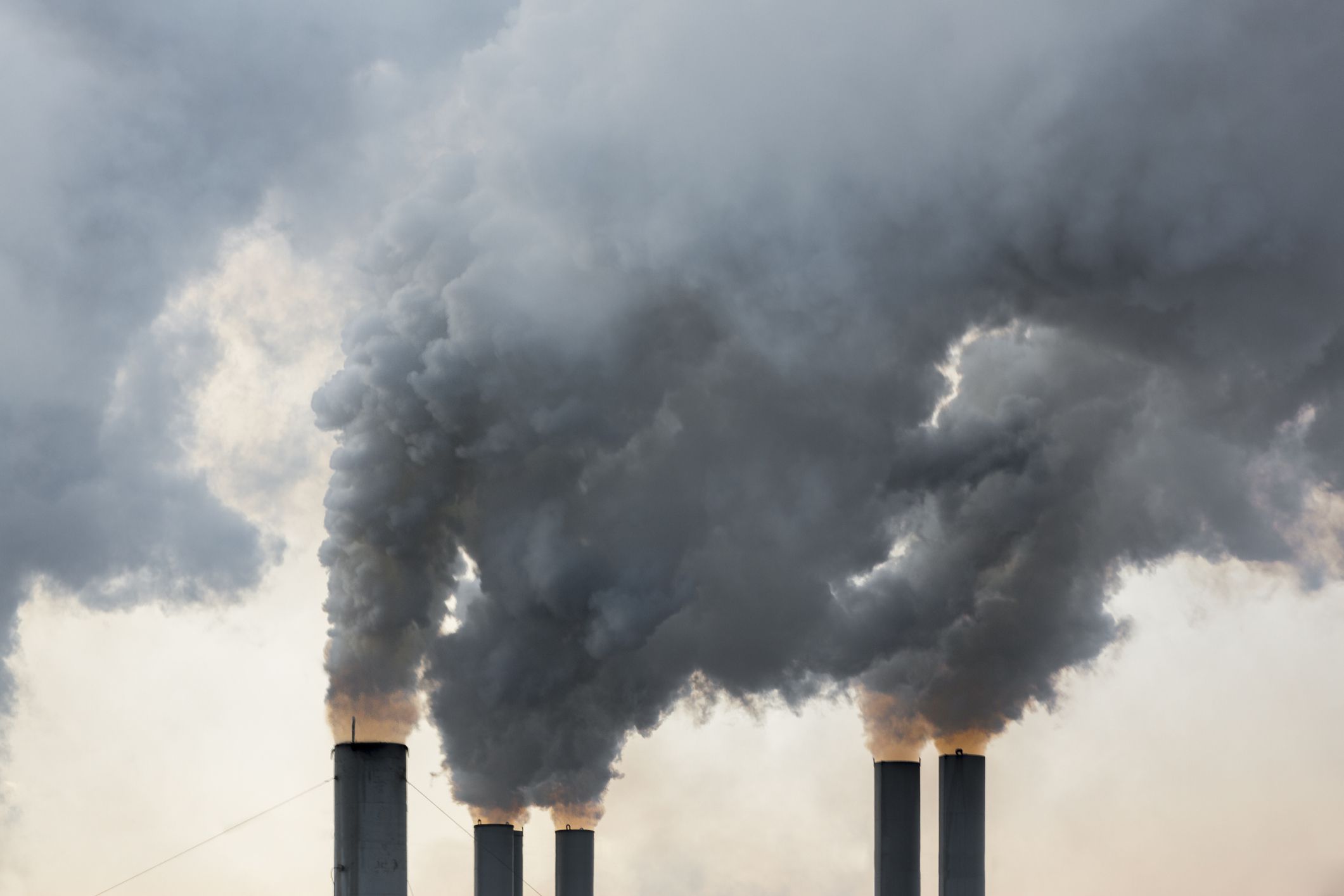
Jeremy Peckham, Research Lead at the AI, Faith and Civil Society Commission
The environmental impact of artificial intelligence (AI) is a double edged sword. On one hand, AI has the potential to enhance energy efficiency across various industries. On the other, the resources required to train and operate AI systems—especially deep learning algorithms—are escalating, leading to substantial energy consumption and environmental consequences.
Increasing energy consumption in AI
AI's rapid advancement has significantly amplified its energy demands. Schwartz et al, report in 2019 in an academic paper, “Green AI”, that “the computations for deep learning research have been doubling every few months, resulting in an estimated 300,000 times increase from 2012 to 2018”. They categorise environmentally unfriendly algorithms as “Red AI”, where the emphasis is on increasing accuracy, for example in Natural Language Processing, rather than efficiency (less energy consumption).
The energy consumption required for training deep learning models is staggering. A 2021 article in IEEE Spectrum provides a striking example: to halve image classification errors, researchers estimated the need for over 500 times the computational resources. This escalating energy demand raises a serious environmental concern. In the pursuit of lowering error rates, for instance, a 5% error rate on the ImageNet dataset—expected by 2025—could cost $100 billion and generate as much carbon emissions as New York City produces in an entire month according to scholars at the University of Massachusetts Amherst. Meanwhile, Google's DeepMind spent $35 million to train its AlphaGo system and OpenAI’s natural language model produced 552 metric tons of CO2 during its training, which is equivalent to the annual emissions of 120 passenger cars.
The IEEE Spectrum article concludes that without rethinking the way we approach deep learning, we may face diminishing returns on progress.
The Role of Green Energy in AI
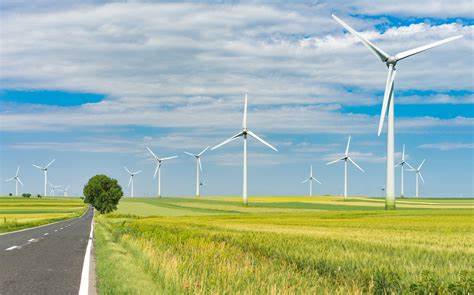
While large tech companies like Google have the financial resources to invest in renewable energy sources, such as wind and solar power, this isn't feasible for every AI company or organisation. In 2021, renewable energy accounted for only 28% of global energy consumption, according to the Renewable Energy Institute. Major AI players may be able to transition their operations to green energy, but smaller companies—particularly those training machine learning (ML) models for specific applications—often lack the necessary resources to do so. As a result, the carbon footprint of AI remains a significant concern.
Specialised chips: A Potential Solution
One promising way to reduce AI's environmental impact is through the use of specialised AI chips. These chips are designed to optimise processing power, potentially cutting the energy requirements of AI operations by up to five times, with even greater efficiencies expected from next-generation chips. As AI technology advances, these chips could play a critical role in reducing energy consumption and improving sustainability across the industry.
Beyond Energy: The Impact on Earth's Resources

The environmental implications of AI extend beyond energy consumption. The production and operation of AI systems rely heavily on scarce earth resources, such as rare metals, which are often mined under environmentally harmful conditions. For example, when consumers purchase devices like Amazon's Echo, they are often unaware of the environmental toll throughout the product’s lifecycle. In 2018, Kate Crawford and Vladan Joler published the essay Anatomy of an AI System, which explored the environmental and human costs of AI devices. Their work, accompanied by a powerful infographic, demonstrated how products like Alexa affect not only energy consumption but also human labour, human rights and scarce earth resources
Conclusion: Balancing AI’s Potential and Environmental Costs
The environmental impact of AI is complex and multifaceted. While AI can undoubtedly lead to more efficient energy systems, its own energy demands—especially for deep learning—are increasing at an unsustainable pace. Efforts to harness AI's potential must consider both its energy consumption and its impact on finite earth resources. By advancing technologies like specialised AI chips and prioritising the use of renewable energy, the AI community can mitigate some of these challenges. However, these efforts need to be scaled globally, with a concerted focus on responsible production and consumption. As AI continues to evolve, its environmental impact must be an integral consideration in its development and application.
Policy Recommendations:
1. Require Transparency in Environmental Impact
Mandate AI companies to disclose energy usage, CO2 emissions, and resource consumption.
2. Encourage Green Energy for AI Operations
Provide subsidies for small AI companies to transition to renewable energy.
3. Support Specialised AI Chips
Fund research into energy-efficient AI hardware and set industry energy efficiency targets.
4. Foster International AI Sustainability Collaboration
Launch global initiatives to share best practices and fund research on AI sustainability.
5. Fund AI Sustainability Research
Increase investment in research for low-energy AI models and carbon-neutral technologies.
References
Jeremy Kahn, A.I.’s carbon footprint is big, but easy to reduce, Google researchers say, Fortune April 22, 2021. [https://fortune.com/2021/04/21/ai-carbon-footprint-reduce-environmental-impact-of-tech-google-research-study/]
https://anatomyof.ai/index.html
https://www.nature.com/articles/s42256-020-0219-9?proof=t
https://mlco2.github.io/impact/
Lacoste et al, Quantifying the Carbon Emissions of Machine Learning, Cornell University, Computers and Society, 2018.[https://arxiv.org/pdf/1910.09700.pdf
Green AI, Schwartz et al, Cornell University, Computers and Society, 2019. [https://arxiv.org/pdf/1907.10597.pdf
Renewable Energy Institute data: https://www.renewable-ei.org/en/statistics/international/